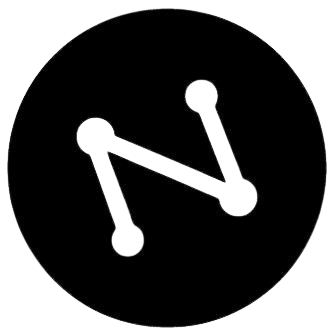
In recent years, predictive modeling has emerged as a transformative tool in healthcare, particularly in clinical management. This technology uses advanced algorithms and large volumes of data to predict future events, allowing healthcare professionals to make more informed and proactive decisions.
One of the most impressive aspects of predictive modeling in healthcare is its ability to identify health risks before they become serious issues. For example, algorithms can analyze patterns in historical patient data to predict risks of chronic conditions, such as diabetes or heart diseases, with remarkable accuracy. This not only improves the quality of patient care but also significantly reduces costs for healthcare institutions by preventing expensive complications and emergency treatments. Additionally, predictive modeling is also helping to optimize resource allocation in hospitals, improving planning and operational efficiency.
Therefore, see in this article how predictive modeling is being integrated into clinical management, its applications, benefits, challenges, and the future of this technology in the healthcare sector.
Before we continue, we need to ask: Are you already familiar with Ninsaúde Clinic? Ninsaúde Clinic is a medical software with an agile and complete schedule, electronic medical records with legal validity, teleconsultation, financial control and much more. Schedule a demonstration or try Ninsaúde Clinic right now!

Fundamentals of Predictive Modeling
Predictive modeling in healthcare involves the use of statistics and machine learning techniques to analyze historical and current data, aiming to make predictions about future events. The data may include electronic medical records, test results, demographic information, and even real-time data on a patient's health status. This approach allows for more informed decision-making and quicker responses to patient needs, potentially leading to better health outcomes.
The integration of tools like 224 Scan, developed by Ninsaúde Software, demonstrates the power of predictive modeling in modern medical practice. Specializing in the interpretation of imaging exams such as X-rays, MRIs, and CT scans, 224 Scan uses artificial intelligence algorithms to quickly analyze large volumes of visual data. Its main goal is to significantly speed up the diagnostic process, reducing the time between conducting an imaging exam and issuing a report to just 60 seconds.
Additionally, 224 Scan offers users the ability to choose where their data is stored globally, ensuring compliance with local and international data protection laws. This capability not only improves the efficiency of the healthcare systems that adopt it but also opens doors for companies from different countries to use this technology without facing legal barriers.
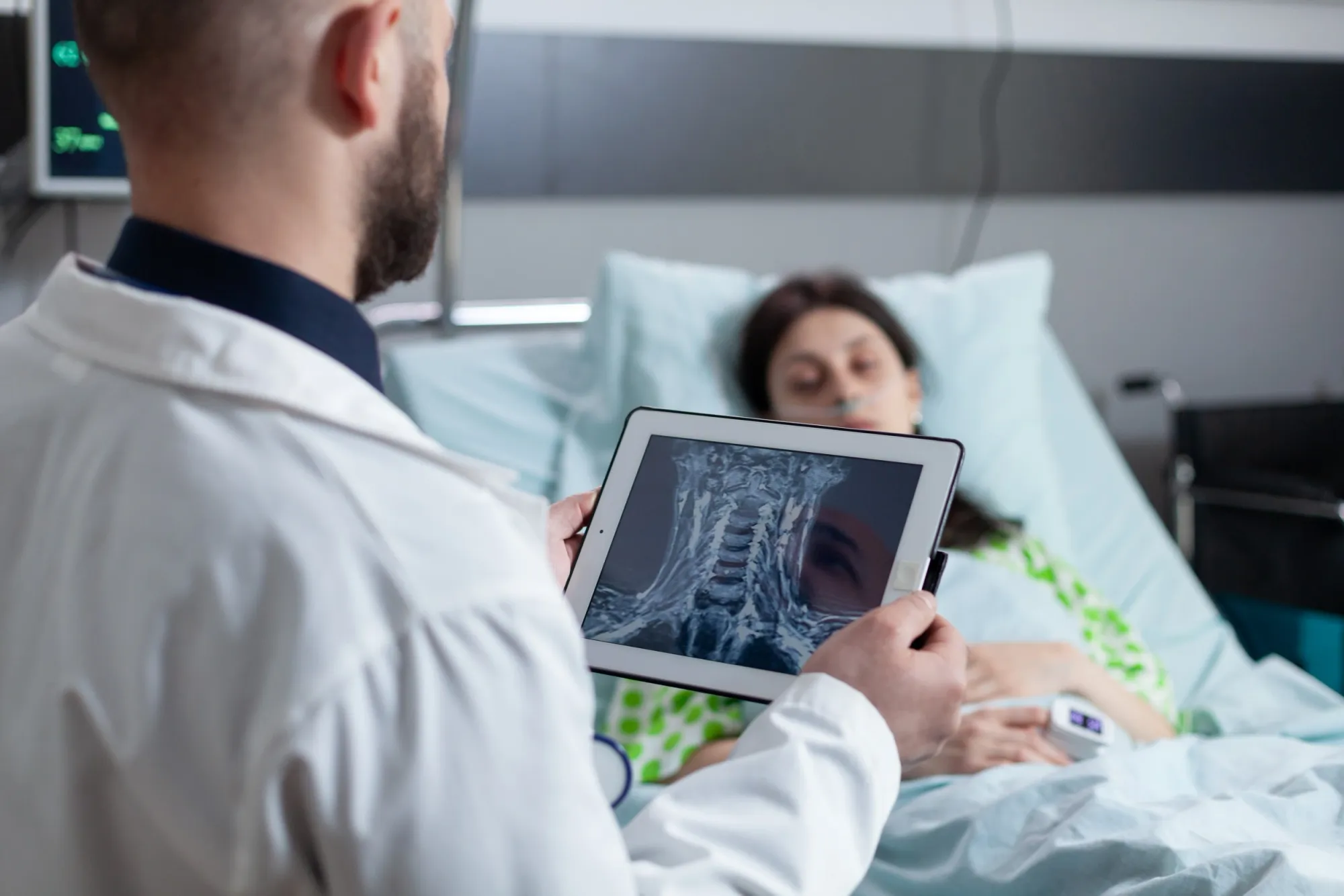
Applications in Clinical Management Using Predictive Modeling and 224 Scan
Prediction of Readmissions: Predictive algorithms are crucial for identifying patients at high risk of hospital readmission. Using historical data and health patterns, these algorithms enable healthcare professionals to carry out specific interventions that can significantly reduce the likelihood of readmission. The integration of tools like 224 Scan can enhance this process by quickly analyzing medical imaging and providing additional insights into conditions that may not be immediately apparent, thus improving the quality of care and contributing to the reduction of operational costs.
Management of Chronic Diseases: Predictive models are essential for monitoring patients with chronic diseases, enabling the prediction of exacerbations and dynamic adaptation of treatments as needed. With technologies like 224 Scan, it's possible to thoroughly analyze medical images to detect subtle changes and indicators of disease progression, allowing proactive adjustments to treatment plans and avoiding acute episodes that require more intensive interventions.
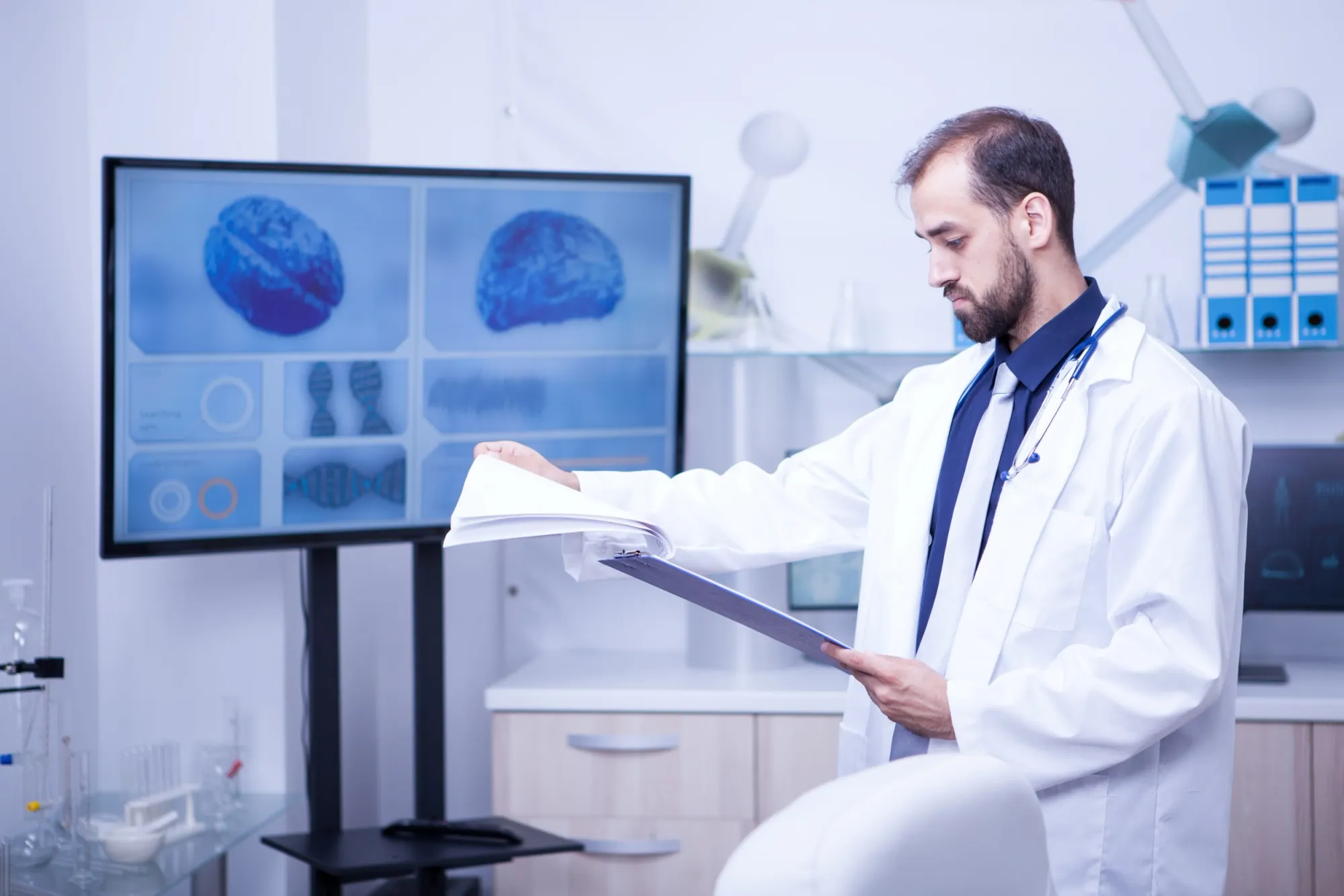
Resource Optimization: Predictive modeling enables the optimization of critical resources such as hospital beds, staff, and medical equipment. By predicting future demand based on hospitalization trends and disease seasonality, hospitals can plan more efficiently, reducing wait times and maximizing the use of their resources. Additionally, systems like 224 Scan can be integrated to produce quick diagnostic reports, facilitating more efficient turnover of hospital beds by reducing diagnostic delays.
Early Detection of Health Conditions: The ability to detect early signs of patient deterioration is one of the greatest advantages of predictive modeling. With algorithms that continuously analyze health data, it is possible to identify at-risk patients before their conditions worsen. 224 Scan complements this process by providing image analyses that identify physical changes before they become critical, enabling early interventions that can be crucial in maintaining health and even saving lives.
Benefits of Predictive Modeling in Clinical Management
The integration of predictive modeling in clinical management offers a range of significant benefits that transform both the operation of healthcare systems and patient experience, including:
Improvement of Care Quality: Accurate predictions provided by predictive modeling enable more timely and personalized interventions. This ability to anticipate health issues and proactively respond to them improves patient outcomes, reducing the incidence of severe complications and enhancing recovery rates. The use of advanced technologies like 224 Scan for medical image interpretation can further increase diagnostic accuracy, providing doctors with detailed information to base their treatment decisions on.
Operational Efficiency: Predictive modeling significantly reduces the time and costs associated with treating unplanned emergencies and unnecessary hospitalizations. By predicting demand peaks and identifying potential emergencies before they occur, hospitals can allocate resources more effectively, avoiding overloads and wastage. Furthermore, tools like 224 Scan help streamline the diagnostic process, reducing waiting times for test results and allowing more efficient patient flow planning.
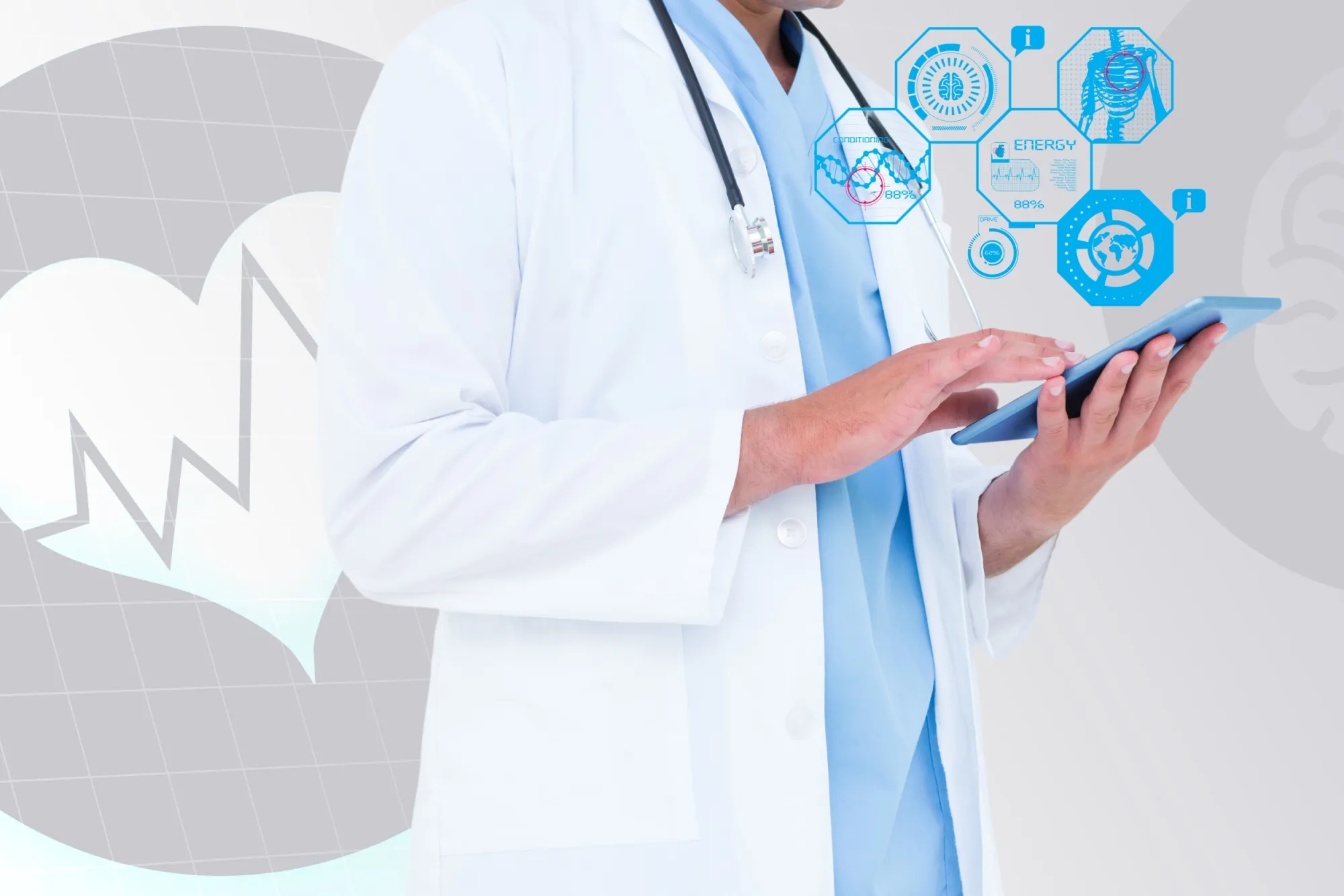
Patient Satisfaction: More proactive health management leads to greater patient satisfaction, resulting from more consistent and preventive care. Patients benefit from a healthcare approach that not only treats illnesses as they arise but actively works to prevent them. Predictive modeling also contributes to more effective communication between doctors and patients, as it provides data that can be used to educate and engage patients in their own care processes, increasing confidence and adherence to proposed treatments.
These benefits highlight how predictive modeling is indispensable for the evolution of modern medicine, making healthcare more adaptive, intelligent, and patient-centered.
Challenges and Ethical Considerations
Despite their numerous benefits, predictive modeling in clinical management presents significant challenges that must be considered. One of the main challenges is data quality, as the accuracy of predictions relies entirely on the quality and completeness of the collected data. Inaccurate or incomplete data can lead to erroneous predictions, adversely affecting treatment decisions and patient outcomes.
Additionally, the use of these technologies raises important ethical concerns, especially related to data privacy. Protecting patient information is crucial, and any failure in this aspect can not only undermine patient trust but also expose the institution to legal risks. Another ethical concern is the potential bias in predictive algorithms, which can result in disparities in the treatment of different groups of patients.
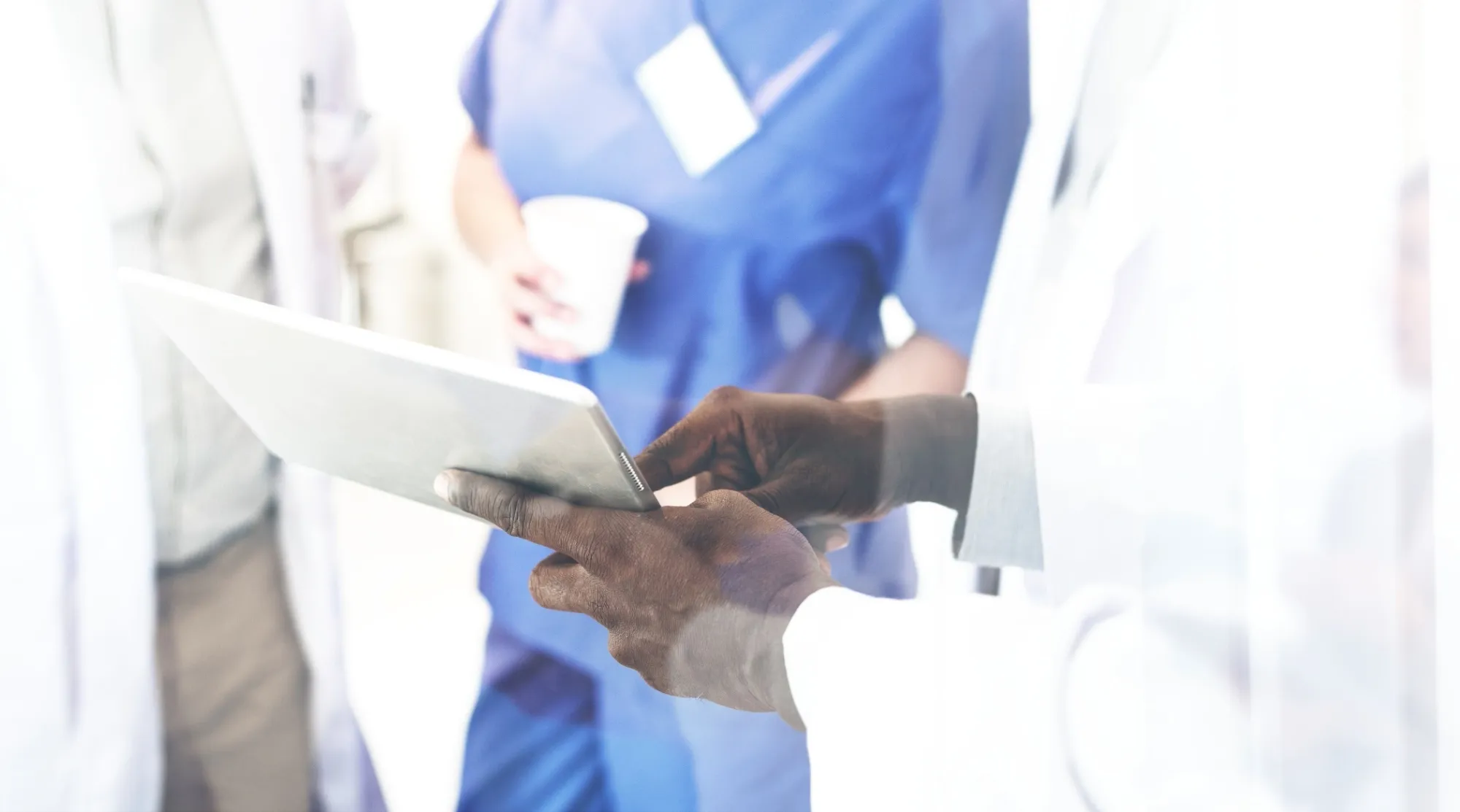
Moreover, the cost and implementation of the necessary infrastructure to support predictive modeling can be prohibitive. The technology requires significant investments in hardware, software, and personnel training, making it an expensive and complex option to implement and maintain, which can be a particularly large obstacle for smaller health institutions or in less resourced regions.
The Future of Predictive Modeling in Clinical Management
The future of predictive modeling in clinical management looks extremely promising and is set to further transform the healthcare field. With the continuous advancement of data technology and analytics, these tools are expected to become not only more sophisticated but also more integrated into daily clinical routines. This includes the implementation of increasingly accurate algorithms capable of processing large volumes of data in real-time, providing faster and more accurate predictions.
The ongoing evolution of algorithms and the increased interconnectivity of healthcare systems through broader and more secure networks will likely expand predictive capabilities, allowing for a more holistic and integrated approach to disease treatment and prevention. This, in turn, will further improve patient care, enabling personalized interventions based on a deep understanding of individual medical histories and emerging patterns in population data.
Additionally, the integration of artificial intelligence, like the 224 Scan tool, into clinical management systems promises to revolutionize how diagnoses are made. These tools can support clinical decision-making, reducing the workload of healthcare professionals and minimizing human errors, which is crucial for treatment effectiveness and patient safety.
Liked the information? Then prepare for a continuous journey of knowledge by following our blog. Are you a health professional and not yet familiar with the benefits of Ninsaúde Clinic? Stay ahead, optimize your processes, and elevate excellence in patient care!
Image Credits for Cover: Image by wavebreakmedia_micro on Freepik